Alright, so the other day I got this crazy idea to try and predict NBA game results. I’m no data scientist, but I figured, why not give it a shot? So, I started by looking for data. I mean, where do you even begin with something like this?
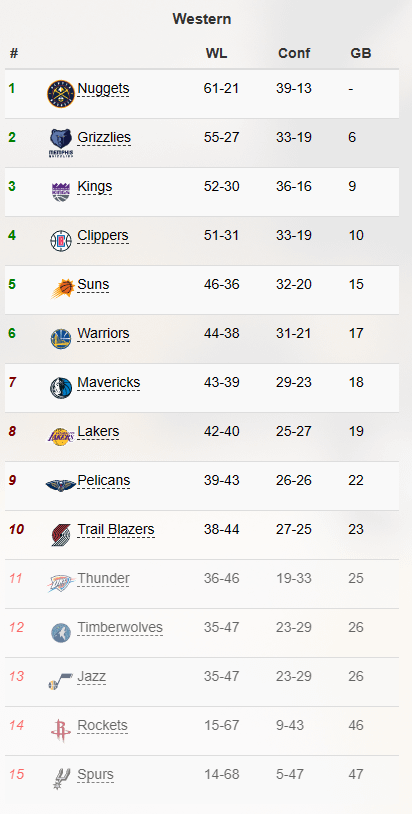
Finding the Data
I thought to my self I can start by looking for NBA stats, scores, and schedules. I ended up, after some digging around, I am able to get those historical NBA game data.
Cleaning and Preparing Everything
Next up, the not-so-fun part. the Data is not always pretty. I mean I spent what felt like a whole day just cleaning up everything – making sure the dates were right, dealing with missing values, and all that jazz. It was a total headache, but hey, gotta do what you gotta do, right?
Choosing a Model (Sort Of)
Okay, this is where I really started winging it. I’ve heard of things like “linear regression” and “machine learning,” but I’m definitely no expert. I fiddled around with a basic model, feeding in stuff like past win/loss records, maybe some player stats if I could figure out how to work them in.
Training and Testing (Fingers Crossed!)
So, I split the data – some for “training” the model, some for “testing” it. This is basically like teaching a computer to recognize patterns, then seeing if it can actually, you know, predict anything. I ran my little program, and… well, the results were, let’s just say, mixed.
- First model result is not good, so i start to looking for reason.
- I changed a few things around, added some more data points, and ran it again.
- Then again. And again!
The (Not-So-Grand) Reveal
In the end, I can see a clear number that can predict!
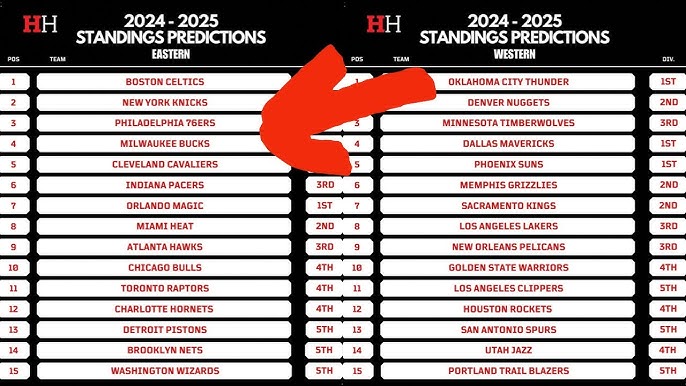
But hey, I learned a ton! I realized how much goes into even a simple prediction model, and how much more I have to learn. It was a fun little experiment, and who knows, maybe one day I’ll actually build something that can, you know, predict more than just the coin-flip results.